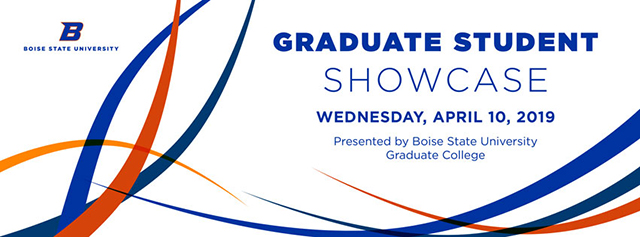
Title of Submission
Learning Behavior of Memristor-Based Neuromorphic Circuits in the Presence of Radiation
Degree Program
Electrical and Computer Engineering, PhD
Major Advisor Name
Kurtis Cantley
Type of Submission
Scholarly Poster
Abstract
In this paper, a feed-forward memristor-based spiking neural network is taught to separate correlated and uncorrelated synapse and learn character inputs using spike-timing-dependent plasticity (STDP). A TiO2 non-linear drift memristor model is used to simulate a neuromorphic circuit with 25 pre- and 1 post-synaptic neuron. During the learning process, memristors are radiated with state-altering radiation and the effect on circuit learning behavior is determined. It is observed that the network recovers when radiation ceases but takes longer to resolve the correlation. Further, at lower but continuous radiation exposure, the circuit may resolve the pattern indefinitely.
Funding Information
The Defense Threat Reduction Agency (DTRA) grant HDTRA1-17-1-0036