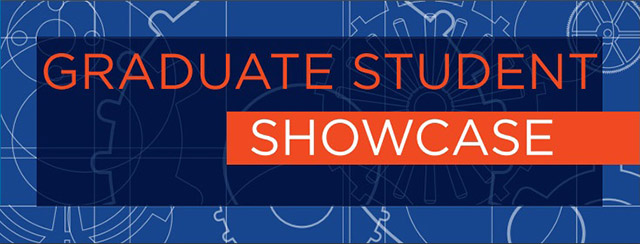
Title of Submission
Degree Program
Computer Science, MS
Major Advisor Name
Michael Ekstrand
Type of Submission
Scholarly Poster
Abstract
Researchers and practitioners often use information retrieval and machine learning metrics (the better the recommendation algorithm can predict users' historical consumption data, the higher the metric score is) to evaluate candidate recommendation algorithms in offline experiments. However, these metrics are biased towards recommenders that favor popular items and provide safe, known recommendations, and they incorrectly assume that items users have not consumed are not relevant. To measure the extent of these problems, we are conducting simulation experiments to quantify the metric error of offline evaluation and investigate the impact of data set parameters on the recommender metric error.