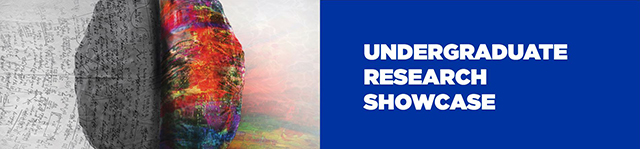
2024 Undergraduate Research Showcase
Using Machine Learning to Model Disease Mortality Rates Across the U.S.
Document Type
Student Presentation
Presentation Date
4-19-2024
Faculty Sponsor
Dr. Liljana Babinkostova and Dr. Marion Scheepers
Abstract
The advancement of machine learning has substantially helped the healthcare industry to identify and predict risk factors with accurate models. This poster intends to utilize machine learning to make a predictive random forest model of different mortality rates across the United States. A random forest model is ideal for this research because of the flexibility and accuracy it has to offer. We plan to show the mathematical concepts that create a random forest algorithm such as decision trees, ensemble methods, and accuracy. The data in this project, U.S. Chronic Disease Indicators (CDI), is a large pool of 124 health indicators per state and is developed by consensus among the CDC, CSTE and NACDD. Focusing on the mortality indicators was the most interesting because the theme was carried across each of the 18 topic groups and our model yielded high accuracy with this focus. This research focuses on the study of various mortality risk prediction models including the regression analysis model.
Recommended Citation
Cobb, Ethan; Babinkostova, Liljana; Scheepers, Marion; Tumir, Max; and Lawrence, Will, "Using Machine Learning to Model Disease Mortality Rates Across the U.S." (2024). 2024 Undergraduate Research Showcase. 119.
https://scholarworks.boisestate.edu/under_showcase_2024/119