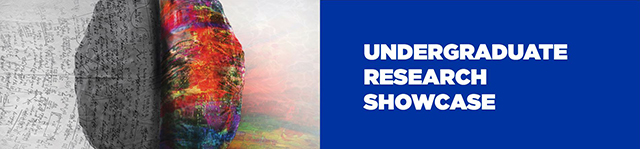
2023 Undergraduate Research Showcase
Document Type
Student Presentation
Presentation Date
4-21-2023
Faculty Sponsor
Dr. Mojtaba Sadegh
Abstract
It is critical to study Suspended Sediment Concentration (SSC) to improve our understanding of the impacts of wildfires, climate, and anthropogenic on the riverine environments. The processes that contribute to SSC occur over a range of spatiotemporal scales making it difficult to continuously measure and monitor. Traditional methods (e.g., field measurement) require significant resources and often lack the spatial and temporal resolution that is needed for important yet smaller-scale systems like streams. In this study, we create an open-source and low-cost method to estimate SSC using remotely sensed data. Our study aims to elucidate if a machine learning (ML) model is capable of estimating SSC from multispectral imagery using a dataset collected in the laboratory. Another objective is to determine which ML model most accurately estimates SSC. Our methods involve testing and comparing two Machine Learning (ML) models: Random Forest and Linear Regression. Preliminary results indicate that the Random Forest model has the capability of extracting the SSC signal from the imagery and providing superb agreement between experimental and ML-generated data (R2=0.99). Further, our results from the Linear Regression model indicate that the relationship between multispectral imagery and SSC is non-linear and requires a more robust ML model (low correlation of R2= -.71). Continuing work will compare these results with other ML models to develop a cross-validated method for widespread usage in SSC detection. This study contributes to a larger framework, and with a validated model we will be able to quantify the effects of SSC on the entire Snake and Columbia River system. Implications of this research include improving our understanding of SSC and detection methods that augment existing methodologies as tools for stakeholders, government agencies, land managers, and citizen scientists.
Recommended Citation
Sheen, Cheyon; Spero, Hannah; Modaresi Rad, Arash; and Sadegh, Mojtaba, "A Step Toward Resolving Spatiotemporal Distribution of Suspended Sediment Concentration Using Remote Sensing" (2023). 2023 Undergraduate Research Showcase. 104.
https://scholarworks.boisestate.edu/under_showcase_2023/104